
From Poetical Science to GANism: A Selective History of the Art in Artificial Intelligence
Artificial Intelligence (AI) generated art has been garnering significant media attention in recent years, but where exactly does the term come from and when exactly did the movement begin? The prehistory of today’s AI art moment could be told in many ways. A lineage can no doubt be drawn between computer art pioneers like Frieder Nake and Vera Molnár, and today’s AI artists like Helena Sarin and Mario Klingemann. But when these artists themselves discuss their inspirations they are just as likely to list mathematicians and engineers as they are their computer art antecedents (Klingemann mentions Marvin Minsky in his interview with Electric Artefacts).
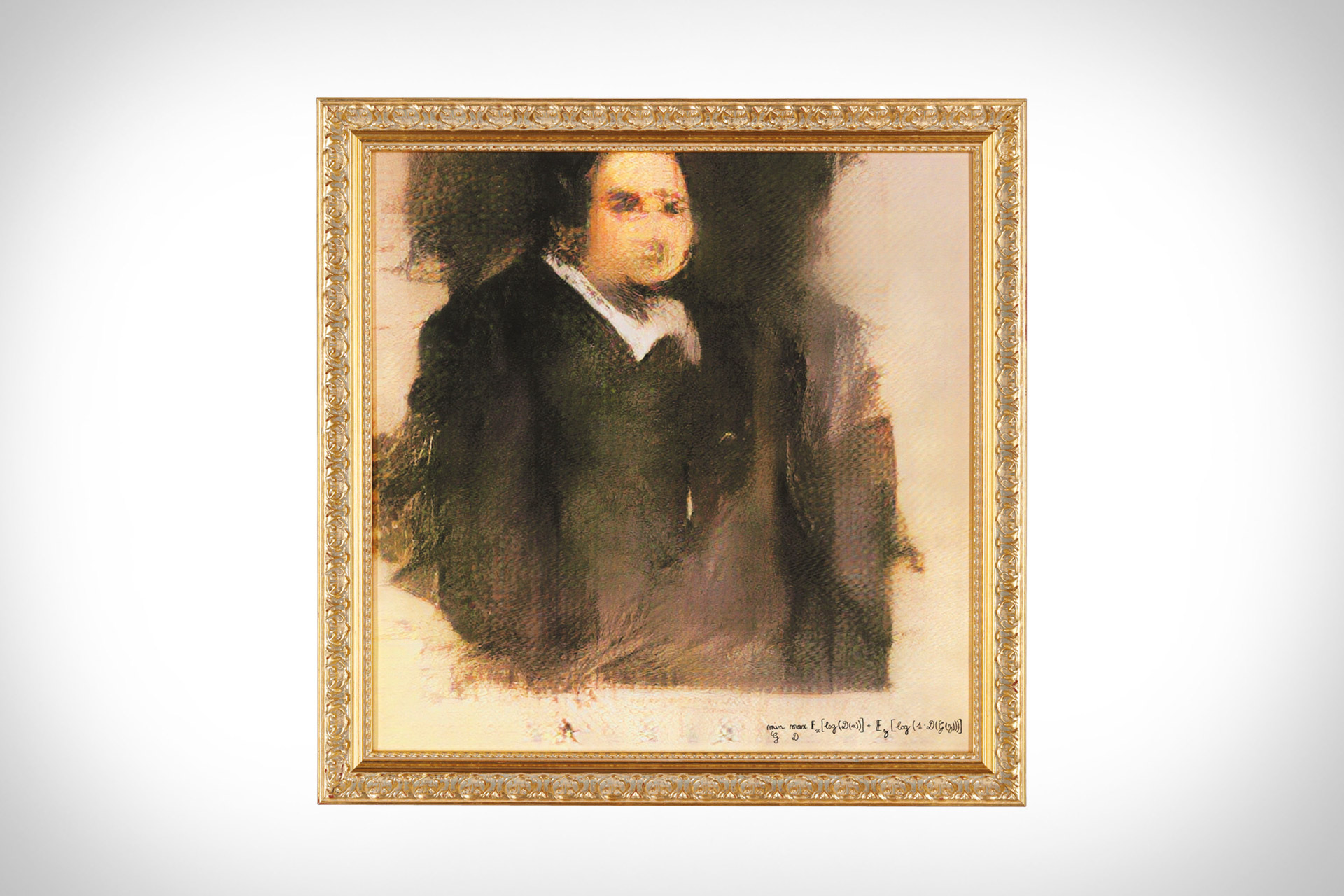
Both Sarin, who has a background in software engineering, and Klingemann, who is something of a self-taught coding wonderkid, are more than competent programmers. And if the controversy surrounding the sale of French collective Obvious’ “Portrait of Edmond Belamy” (2018) tells us anything, it is that to the nascent AI-art community, the credit for creating an artwork should remain at least partly with the person who programmed the algorithm behind it.

Shifting focus to the technical, rather than the aesthetic dimensions of AI art, reveals that the history of computing is as much a part of art history as the various movements in painting and sculpture that signalled the arrival of modernity towards the end of the nineteenth century.
Ada Lovelace, the mother of computer science, prefigured twentieth century developments in machinic creativity when she speculated that computers would eventually be capable of composing music. The idea behind Lovelace’s speculation was simple. Early computers were limited to simple arithmetic operations because the rules of arithmetic were already well understood. She coined the phrase “poetical science” to describe her approach to mathematics and engineering and believed that a machine could theoretically operate on any system analogous to the set of real numbers, given a similarly established set of rules for how its component should interact.
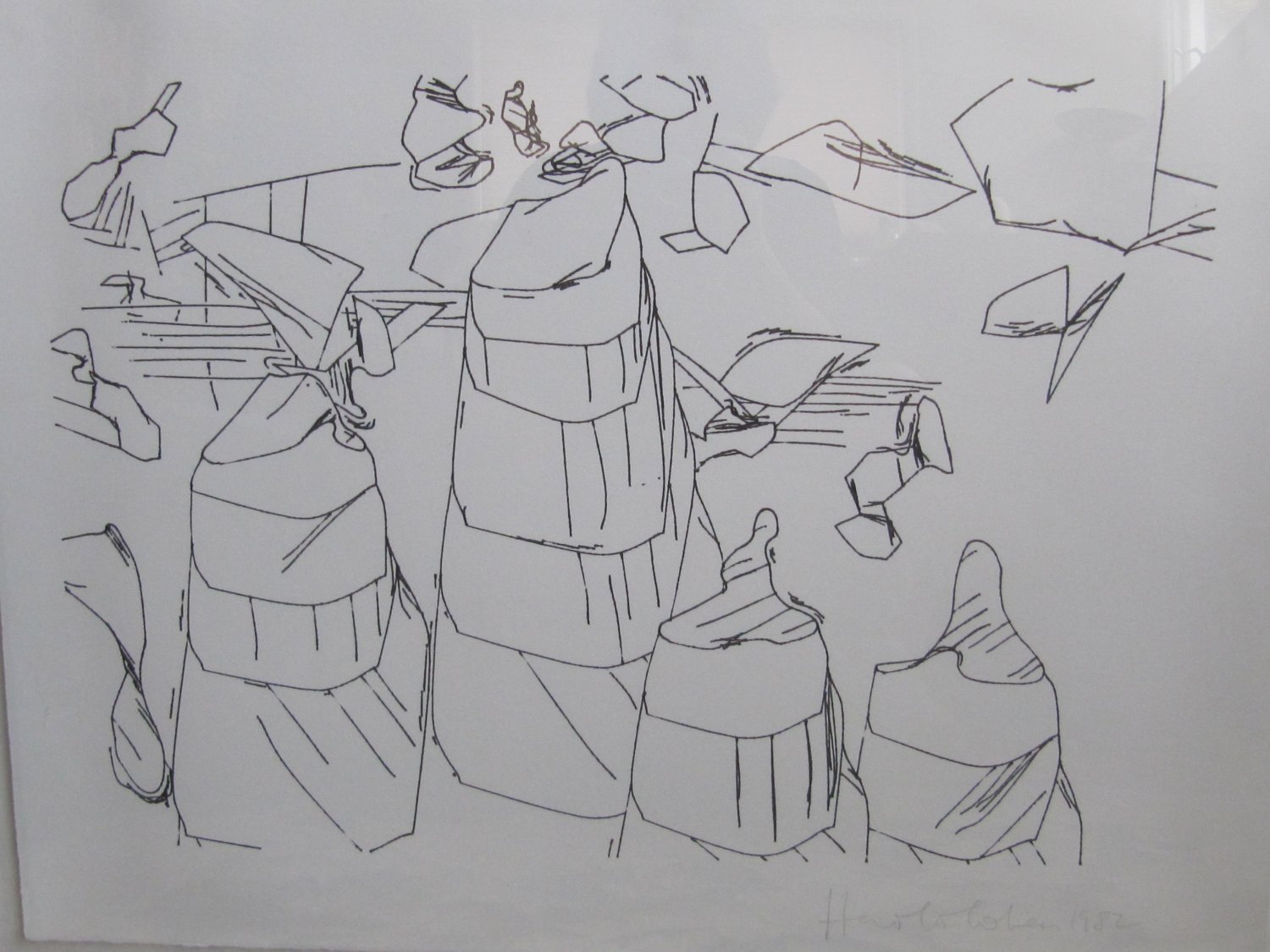
Lovelace’s intuition was the same one that inspired Harold Cohen to start creating the first AI art with his program AARON in 1973. A painter by trade, when Cohen first encountered computers he immediately saw an analogy between the way he painted, and the way code worked. Just as his paintings were the result of standards he instinctively followed, he soon realized the potential of computer programs to apply a set of standards and norms to the challenge of generating art. But more than any of his contemporaries working with computers, Cohen was fascinated by the capacity of the program to constantly surprise him.

The evolution of AARON from its inception in the early 1970s charts the slow but steady rise of artificial intelligence in the proceeding decades. In a period that gets referred to as the AI winter for its lack of significant progress in AI research, Cohen incrementally improved his program, whose first outputs were monochromatic child-like line drawings, but by the time of his death, could generate highly figurative images with foreground, background, and multiple colours.
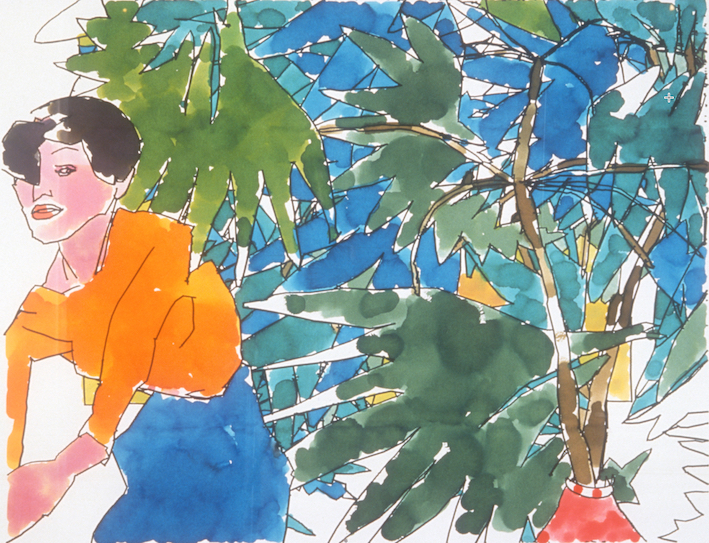
While AARON’s output could be both beautiful and surprising, it was still formulaic, and each new style that the program developed was the result of new instructions given to it by Cohen. First hypothesized in 1943, it would take advances in artificial neural networks (ANN) in the twenty-first century before programs outputting AI art would begin to develop their own style with minimal prompting.
The ability of neural networks to generate images has been a happy consequence of research into image recognition. The late twentieth-century boom in machine learning fed into an explosion of ANN research from the mid-nineties onwards. But despite significant leaps represented by new approaches to ANNs, the datasets that these networks trained themselves on remained a limiting factor in their application to image recognition. It was with this problem in mind that Fei-Fei Li, a researcher at Stanford university, set about developing the largest ever collection of human-categorized images. What resulted from the project was ImageNet, a free database of 14 million images labelled by tens of thousands of crowdsourced workers that Li released in 2009.
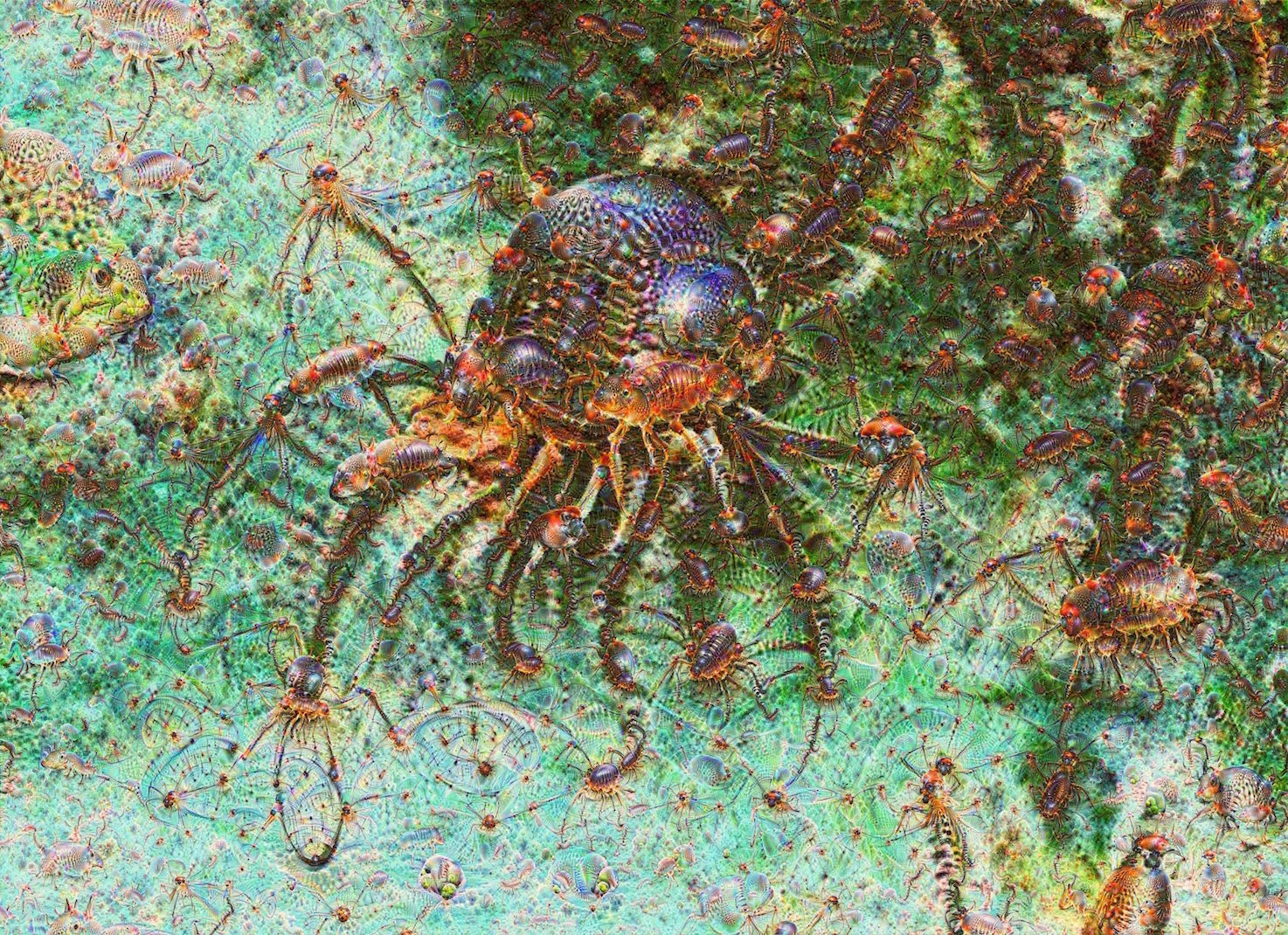
Arguably the catalyst for the current AI boom the world is experiencing, ImageNet quickly evolved into an annual competition to see which algorithms could identify objects with the lowest error rate. It was Google engineer Alexander Mordvintsev’s entry to this competition that would go on to be released as the DeepDream computer vision program a year later. Although initially developed with the aim of recognizing images in order to automatically classify them, when the process is reversed, DeepDream becomes a powerful tool for artistic expression.
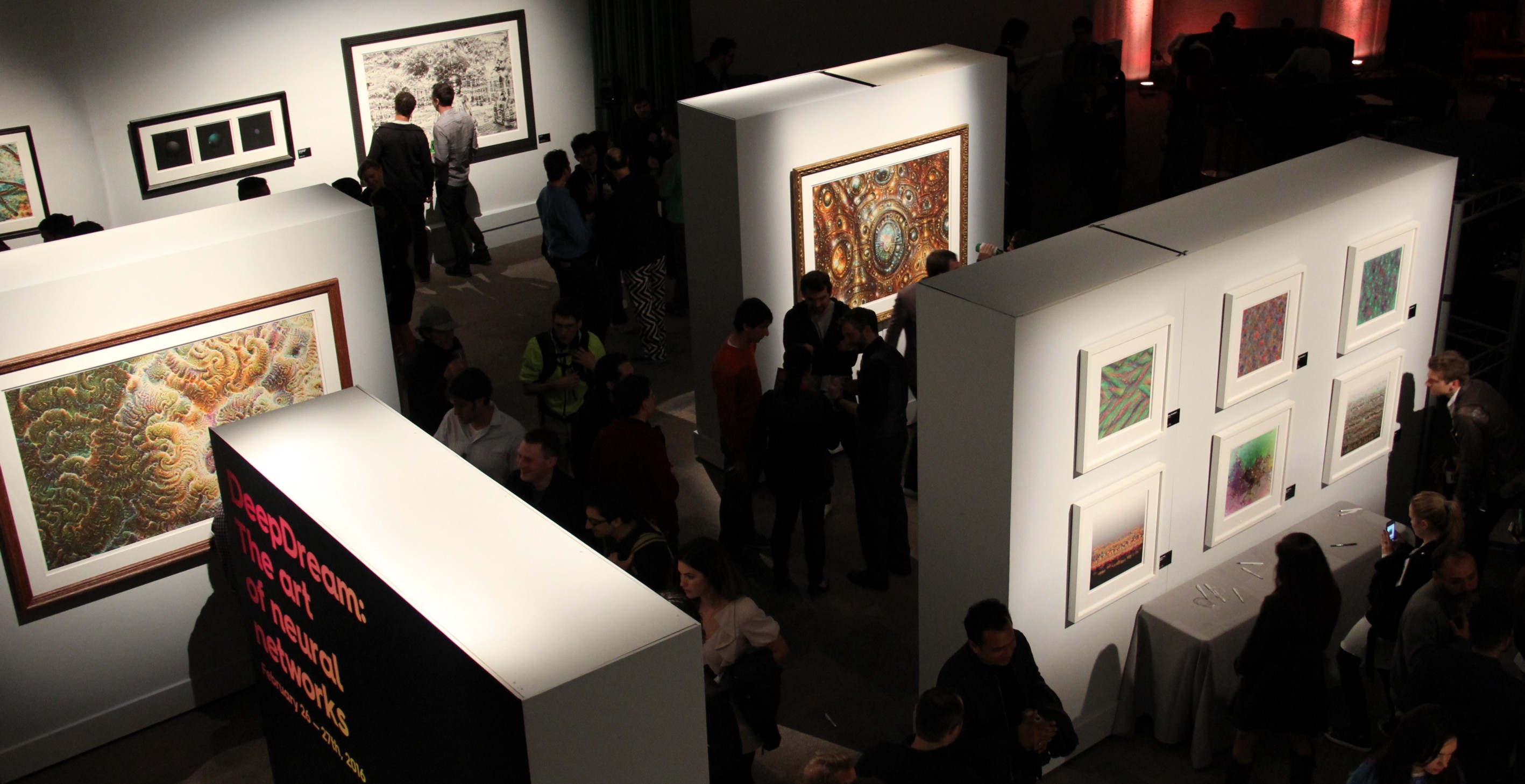
With a seminal exhibition of DeepDream art in 2016 (above), the now instantly recognizable early works—heavy on eyes, fur-like textures, and trippy, hybrid animal and architectural forms—soon caught the public imagination. Thanks to DeepDream being open-source, and the concurrent development of Neural Style Transfer, the years since 2016 have seen an increase in the accessibility of machine learning techniques for both amateur and professional artists.
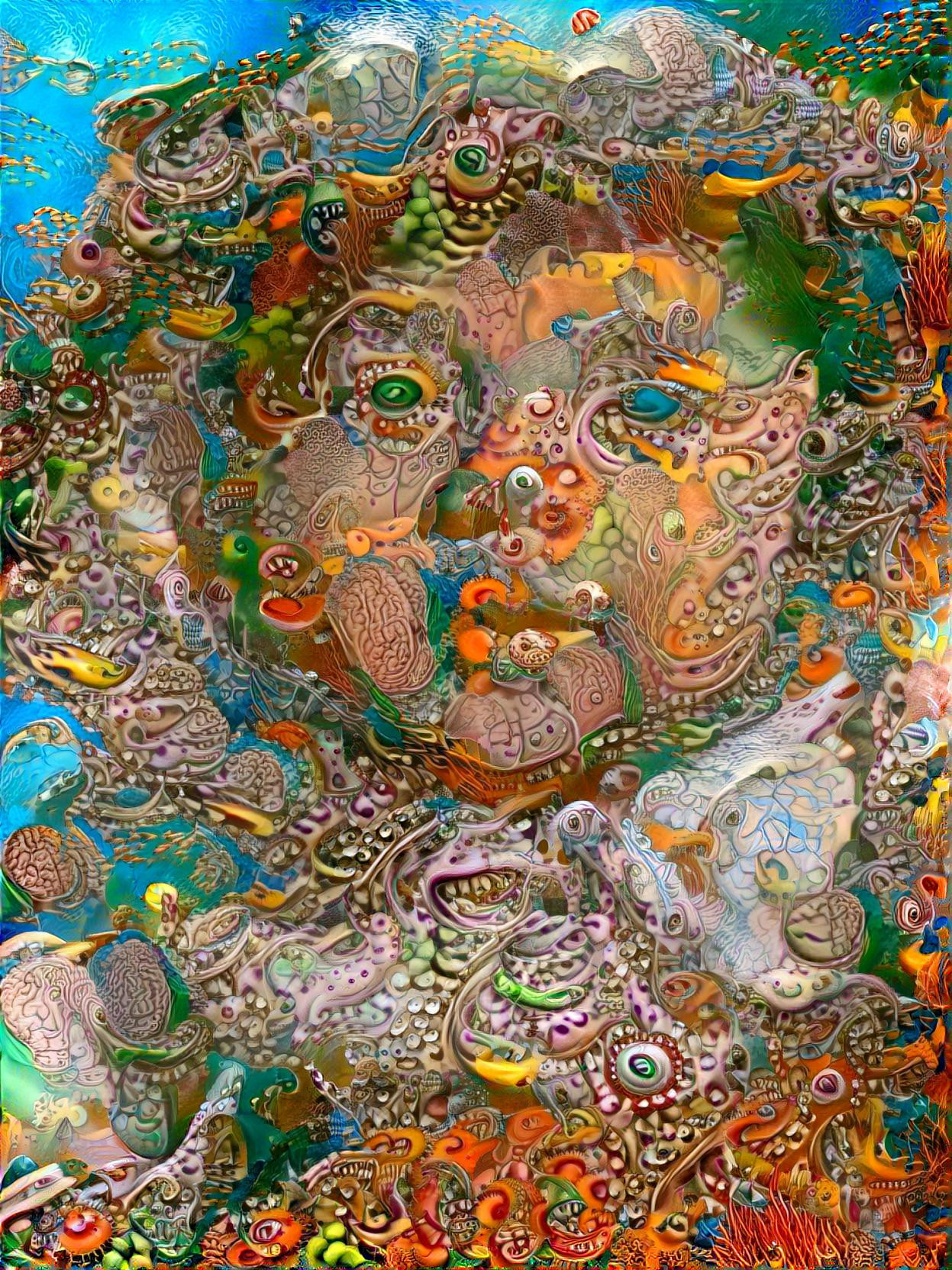
Another AI innovation that has been readily adopted by various creatives is the Generative Adversarial Network (GAN). Such has been their impact on the world of AI art that in 2019 The Verge wrote that “the images created by GANs have become the defining look of contemporary AI art”. The term GANism has even been coined to refer to a new genre that wields the adversarial network as its paintbrush. Yet just as some of the earliest work to utilize DeepDream already appears cliché, as ever, artists would need to push the boundaries of the new medium if they wanted to remain interesting.
One approach to GAN art that has yielded interesting results has been diversification of the kinds of datasets used to train the networks. Mario Klingemann has trained his AI models on various libraries that include photos from yearbooks, encyclopaedia entries, and paintings by the old masters of European portraiture. He also frequently uses chains of different GANs to create his artworks. Likewise, Helena Sarin has developed a completely original style of “neural bricolage” by training networks on her own drawings, paintings, and photographs.

Transfigured into moving images, often by placing AI into a continuous feedback loop between its output and input, contemporary neural network art recalls the 1960s’ interest in video-feedback associated with Nam June Paik and the New York school of psychedelic art. Just as those videographic pioneers immersed their audiences in a multimedia, audio-visual experience, artists working with AI today have combined sound and image to powerful effect.
Isabella Salas’ “Small Flower Bits” sets a tantalising floral morphology to the dreamy soundscape of Henry Thr Miller’s Small Bits. Typical of neural art, just as something recognisable begins to emerge in the piece—a not-quite-fuchsia, or an almost-orchid—it has moved on, leaving our cognizance grasping at something that was never there.

In another work, “Ocean Within”, Salas fed her GAN photos of endangered coral reefs around the world, while artists like Juan Covelli and Hexorcismos have moved towards decolonising AI by engaging their models with indigenous visual cultures and histories. Thus, as AI art matures, the question of ownership transforms from an aesthetic judgement into a critical one. Artists from Cohen to Klingemann have argued that attributing authorship to code is a moot point because code itself is authored, but the question of who takes responsibility for the way new technologies shape the world is still of pressing concern.

In a techno-political environment of algorithmic governmentality, deepfakes, automated decision making, and machine-learnt bias all point towards the growing problems society faces as AI becomes a part of our world. In the 1930s, Walter Benjamin argued that the power of technologically informed art is that it actively shapes relationships of production, providing alternative visions of how technology can be used. The same is no less true today, and as it did then, art stands at the forefront of contemporary debates over what specific technologies mean, and how they should be used. Beyond GANism, artists like Pierre Huyghe and Martine Syms have propelled AI in a more conceptual, more critical direction. In this story, where we go from here no-one can predict, but we can be certain that the kinds of art discussed here will have profound implications in the years to come.
Join us Feb 9th for an online opening of our next show focused on art made using artificial intelligence. Sign up for the Newsletter to get your invite here.
More details here.
Cover image by Chris Kore, still from Almnesia. Memory 0.2
